A Beginners Guide to Data, Noise and Uncertainty
We will answer the questions:
-
What is ‘noise’ in relation to data?
-
What is uncertainty?
-
How does uncertainty change when we collect data?
What is ‘noise’ in relation to data?
The data you acquire in an experiment, observational study, or retrospective analysis of previous work are the numbers you will ultimately insert into an analysis.
-
Data, unfortunately, are imperfect.
-
that is, they are 'noisy'
-
-
Let’s explain this concept in terms of the literal meaning of the word.
-
When you are having a conversation at a crowded party, you will have more trouble understanding what is said to you than if you were having the same conversation in a secluded pine forest.
-
The difference between these two scenarios is that the party environment is noisy. This ambient noise is literally added (in the sense of superposition of waves) to the sounds you want to hear, corrupting those sounds and therefore making them harder to understand.
-
This can be contrasted with a ‘noiseless’ or uncorrupted set of sounds that you would have ideally been sent.
-
The noisy spoken message in the party environment is, in most cases, analogous to the data that you analyze.
-
You can imagine that there is a noiseless dataset that consists only of the sounds representing the intended signal, and this noiseless dataset is then corrupted by noise from various sources (the sounds made by other partygoers).
-
The more noise that corrupts that noiseless dataset, the harder it is to correctly identify the underlying message.
-
Noise in the data leads to uncertainty in the underlying message.
-
Noise in the data does NOT lead to uncertainty about the data.
-
In the noisy environment, the sounds entering your auditory canal are the noisy data that you will have to interpret.
-
Those data are not uncertain. They are the actual sound waves that arrived at your ear.
-
Your uncertainty regards whether those data represent one underlying signal vs. another (whether the sound waves arriving at your ear are the noise-corrupted sounds that represent one word vs. another word)
-
Your uncertainty is NOT about your dataset (which sound waves actually arrived at your ear), but rather about the signal they represent (which words were spoken to you).
-
What is uncertainty?
-
The term uncertainty refers to the possibility that there are differences between our information regarding facts, and the facts themselves.
- When uncertainty is low, we believe that our best guesses regarding facts are quite accurate
-
If uncertainty were high, we would instead have less confidence in the accuracy of our best guesses regarding those facts
In both cases, uncertainty relates to a belief. That belief might be correct or incorrect. It is our job as scientists to bring our beliefs into register with reality by collecting and analyzing data.
How does uncertainty change when we collect data?
To understand this relationship, we should ask ourselves: Why am I collecting data?
We will assume here that your goal is to make a measurement.
Before we collect data, we must have a model of the measurement.
The simplest such model is that used for temperature measurement, the ‘simple additive noise’ model:


which treats thermometer readings (the data, x) as a noisy (i.e. corrupted by noise, ) replica of the true temperature ( ).
This is exactly analogous to the additive noise-corruption in the cocktail party scenario
-
In other words, there is a true temperature, and you infer that temperature by observing data.
-
Each datum (x) you observe is not exactly equal to the temperature
-
it is related to the true temperature ( ) through the model
-
in this model, each datum is a noise-corrupted (the addition of ) version of the value
-
-
We must also model the noise, .
In this temperature measurement scenario, one typically models the probability distribution over the noise term as a zero-mean Gaussian distribution with standard deviation, .
Thus, uncertainty enters this measurement scenario because you don’t know the value of the noise-sample, , that is corrupting the signal, , to produce your data-sample, x.
-
Thus, when you observe a thermometer reading of 99.3˚F
-
is this because the true body temperature is 99.3˚ (i.e., =0˚)?
-
Or is this because the true temperature is 98.6˚, and =0.7˚?
-
Thus the noise-corruption of your dataset makes it uncertain which true signal (value of ) produced your dataset.
In particular, for a given thermometer reading,
there are two unknowns:
-
the value of the true signal ( )
-
this is the actual body temperature
-
-
the particular noise sample ( ) that is corrupting that signal in data
-
this is the noise corrupting your thermometer reading
-
The connection between the distribution describing possible noise samples (the sampling distribution, Fig. 1) and potential underlying signals that might be contained in your noisy dataset is the likelihood, written:
Notice that the object of the probability statement, while usually representing an unknown quantity, is here the known value of the dataset, x.
That’s why the likelihood is written:
to emphasize that the likelihood is a function of the unknown value of the signal ( ).
-
It tells us about the values that are more or less consistent with the observed dataset.
-
Let’s say there are two thermometer readings in our dataset:
-
Let’s further suppose that the sampling distribution describing possible noise samples is:
This means that the likelihood is:
because (from the definition of the data),
We can now ask:
- what is the likelihood of the signal having been 98.6˚?
- what is the likelihood of the signal having been 98.8˚?
- what about 99˚?
- what about 100˚?
If we make this computation by holding the data constant at the observed values, , and varying the possible values of the unknown parameter, we obtain Fig. 2a.
Now let’s observe the thermometer a few more times, expanding your dataset to:
We can repeat the same procedure
- keep the dataset constant based on these 7 thermometer readings
- compute the likelihood for a range of possible underlying signal values ( )
This procedure yields the new likelihood function in Fig. 2b.
We can see that two things happened
- the location shifted a bit toward the true simulated value at 98.6˚F
- the width of the likelihood decreases substantially
Reduction in the width of the likelihood seen between the (a) and (b) panels of Fig. 2 tells us that measurement uncertainty is being reduced.
To end, we should take a step back and ask ourselves what these likelihoods mean and don’t mean:
-
By reducing the uncertainty, do we know that the location/mean will stop changing?
-
No, the mean will continue to change as new data are acquired, but the rate of change will (on average) decrease, because means are always able to change, although introducing a new datum of a given size will have more of an affect on the mean when there are fewer previous data in the dataset.
-
-
Do reduced widths of the likelihoods computed from larger datasets tell us that the true value of body temperature must be within a smaller and smaller range of values?
-
Actually, no.
-
The range of possible body temperatures does not change, regardless of how thin the likelihood becomes.
-
Rather, we become more and more confident in the location, but generally do not become completely certain.
-
Thus, the true temperature is sometimes found to be outside the main body of the likelihood (e.g., temperature at 101 when the likelihood is that of Fig. 2b).
-
Narrower and narrower likelihoods simply make us more confident that large deviations between the peak of the likelihood and the true value are unlikely
-
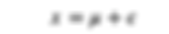

























